A/B testing is a critical and of-overlooked element of data science at high-flying tech firms like Google, Netflix, and Uber, but it can be difficult to set up a testing infrastructure. Now you can implement A/B testing with Eppo, an experimentation service founded by a former Airbnb data scientist.
Chetan Sharma, the CEO and founder of Eppo, remembers the early days of Airbnb, before the company made it big and became the poster child for the power of transforming entire industries with data-driven innovation. Even back in 2012, the company’s ethos around continuous experimentation was beginning to take shape.
“For anyone who was there at the time, it was abundantly clear that experimentation was going to turn the corner,” says Sharma, who was the fourth data scientist hired at the firm. “That’s what got people to start thinking about metrics and really just unleashed this entrepreneurial culture that let people take risks, try stuff out.”
Experimentation held a powerful sway in the vacation rental startup. Instead of holding a lot of meetings and getting sign-off from the corporate hierarchy to make some change in the product, why not just implement the change in a control group and see what the results were before rolling it out to the masses? It’s the ultimate in empirical thinking and a driver of fortunes in the data economy, but it wasn’t always so easy at Airbnb.
“Some of our biggest metric lifts were things that had some internal resistance,” Sharma, who goes by Che, told Datanami today. “I was there for five years, and by far the most successful, impactful experiment we ever ran was when you click on Airbnb’s listing, it opens in a new tab.
That seemingly innocuous little change in the application’s behavior boosted bookings by 3% to 4%, Sharma said. “A huge amount,” he said. But without being empirical and gathering the data through a properly conducted experiment, Airbnb’s upper management may not have believed it was true.
Sometimes, A/B testing tells you what you should do. And other times, it tells you what you should not do. For example, back in 2013, Sharma recalled how Airbnb was investing large sums of money to create glossy neighborhood guides. The project, which was spearheaded by the head of product, would tell guests where the hottest or most glamorous places to eat, drink, and be seen in certain locales.
It was all put together in a very slick manner. However, it may have worked a little too well, because A/B testing showed that it actually hurt bookings, Sharma said. “People get started trying to book at Airbnb and then they end up reading pages and they get distracted,” he said.
Ubiquitous A/B Testing
The casual observer may not see it, but the Airbnbs of the world are constantly running A/B tests to optimize all elements of their businesses. They run so many tests that the experimentation mindset becomes ingrained in the culture of the company.
“Every single thing that ships at these companies goes through an experiment, across the board,” Sharma said. “It’s one of those secrets lying in plain sight.”
A/B testing touches multiple personas within a company, but the data team ultimately is responsible for ensuring the metrics are correct, that the data pipelines don’t break, that the tests are conducted in a statistically valid manner, and that the results are communicated clearly.
Accuracy is precision are paramount in any experimental endeavor, and it’s no different in A/B testing. While the architecture that most companies use to run A/B tests is not too complex, Sharma said, there is a certain depth to each of the components that must be done correctly.
“You’ve got in-app randomization, data pipelines, stats, reporting. There are a lot of pieces that have to work in harmony, so it ends up being quite a lot,” he said. “Even at companies that have built it in-house, either their experimentation culture sputters a lot and doesn’t quite take off, or there’s a serious headcount committed to it. There’s a lot of people building and maintaining these things.”
A/B @ Eppo
Eppo’s A/B experimentation platform runs in the public cloud. The product sits atop data warehouses like Snowflake, AWS’s Red Shift, and Google Cloud BigQuery. It can be used to run A/B experiments on anything in a business, from the color or placement of a button to the assortment of an order in a supply chain setting. Mostly, it’s used with data products and user interfaces, Sharma said.
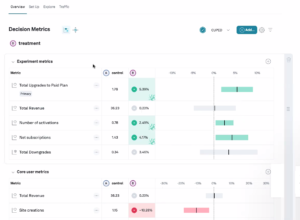
Eppo’s software is designed to enable users to run A/B tests wtihout being statistical experts (Image courtesy Eppo)
Users start with the product by defining the business metrics that customers want to impact, such as revenue or sales. That’s done using SQL. Once those SQL snippets have been set up in a repeatable library, then data engineers or scientists can use them to design experiments to test how metrics are impacted by product changes upstream.
There’s a lot of statistical work that goes into A/B testing, but Eppo shields most users from needing a deep statistical background to use it.
“You often have a finite set of experts, mostly sitting in the data team, who understand the metrics, who understand stats. We need to scale them out,” Sharma said. “Everything is made to be very intuitive.”
For example, instead of giving the result of an obscure power analysis that many data scientists may not know, Eppo presents users with a progress bar showing how much more data is necessary to come to a statistically relevant conclusion for a given experiment.
Normally, A/B tests requires a certain amount of time to work. A month and a half or two months are often cited as minimum timespan to be sure that a representative sample has been drawn from the user base. But Eppo uses the latest statistical methods that can cut down on the amount of time that an experiment must run before giving valid results.
One of those is sequential analysis. “It basically gives you always valid results. Every time you look at an experiment, it will be statistically sound,” Sharma said.
Another is CUPED variance reduction, which Airbnb uses for all of its experiment. This approach can cut the time requirement by 20% to 65%, Sharma said. “Weeks of time you get back,” he said.
A Culture of Experimentation
Eppo, which was founded in 2022, today announced a Series A round worth $16 million. Led by Menlo Ventures, the round puts Eppo’s total funding at $19.5 million following a previously unannounced $3.5 million seed round.
The company hopes to streamline the development of A/B testing, both for companies that are new to A/B testing as well as for companies that have experience with it and are ready to take it to the next level. In either case, the pursuit of a pervasive company-wide culture of experimentation is the ultimate goal, Sharma said.
“Data teams are actually experimentation teams. It’s kind of funny, given the level of consequence of this stuff, that there aren’t really any great commercial tools yet,” he said. “You don’t really know what product changes are going to lead to the biggest results, and so the best benefits happen when you [test] it comprehensively. Who would have thought that the booking the new tab experiment was going to be the biggest metric mover over the machine learning model that took three months to build? You don’t really know that stuff before you measure it.”
Related Items:
AI Bias Problem Needs More Academic Rigor, Less Hype
ML Needs Separate Dev and Ops Teams, Datatron Says
Using A/B Testing to Improve Your Business
The post A/B Test Like You’re Airbnb appeared first on Datanami.
0 Commentaires