How the term “artificial intelligence” is defined and whether there can even be a canonical definition at all is currently a matter of debate in information technology, neuroscience, philosophy, and linguistics. Commonly understood, the term “artificial intelligence” is used to refer to a machine being able to do things that would demand intelligence from a human. This level of artificial intelligence would, however, generally be considered “strong AI” – as opposed to the weak rendition.
With strong AI, a machine would think on its own and act on its own, exhibiting a level of independence which is, to date, not to be found outside of science fiction. Weak artificial intelligence, on the other hand, simply refers to the application of human-like skills (think text recognition, image recognition, and speech recognition) to machines. Weak AI has developed at a rapid pace in recent years and is already commonly used in everyday life. Because we are addressing its use in invoice processing and other business processes, the term “AI” in this article is always meant as a reference to weak AI.
The umbrella term AI covers a number of subsets of different technologies, including machine learning (and a particular type of machine learning, deep learning), neural networks, and natural language processing. In practice, however, the term AI as it is used today is often associated with only one of these subtypes, machine learning. This refers to algorithms that can learn independently from data.
Due to the enormous computing capacities of present-day computers, it is possible to search through vast amounts of data in seconds. Additionally, computers’ ability to detect patterns has been shown to be far superior to that of humans. These two vectors, speed and pattern detection, enable a computer system to improve results and find solutions faster and more accurately than a human can. It can intelligently link data, capture correlations and make predictions with a certain amount of probability.
AI Has Arrived at the Workplace
The most recent developments in deep learning advance this trend further: Less data is needed to create equally good search results. “Few shot learning” and “transfer learning” are recent advances in this area. Toolsets are also improving, requiring fewer skills when using software with AI algorithms. At the same time, the cost of these kinds of technologies continues to decline, and we are seeing a growing acceptance of AI for a broad spectrum of practical application.
Due to advancing digitalization in recent years, AI has outgrown the experimental laboratories which it once called home. Now, widely varied applications can be found, both in business and in the private sphere. Chatbots, product recommendations in online retail, and risk analysis for fraud protection are just a few of these applications. At the same time, the use of AI opens up new opportunities for companies to minimize errors and to control business processes such as invoice processing more efficiently.
Capture: the Achilles Heel of Invoice Processing
AI offers great potential when it comes to automated invoice processing. In analog processes, capturing and validating incoming invoices, transferring invoice data into the ERP system, and routing invoice documents into an approval process were still largely manual activities. The negative side effects of doing all this “by hand” become particularly apparent with large document volumes: The monotony of manual data entry and checking can introduce errors, and work easily expands to the time allotted. Paper invoices get lost or go missing, cycle times become longer, and opportunities to take advantage of discounts and rebates are lost.
This is where the use of automated invoice capture software can minimize errors and accelerate processes. Indeed, when AI is involved, the gain in efficiency even grows with the volume of documents processed. Not only does software make data entry easier and minimize the need for manual processing of paper invoices, automating the capture step optimizes AI functionality by generating data. As documents are digitized, the quality of the output is validated. With AI, this is the basis of a continuous improvement process.
Common practice in data extraction has involved the use of predefined templates to determine where in the document certain data is captured. An invoice number, for example, is assumed to be located in the header data area, item data in the middle area, and information on the vendor both in the sender address and, together with account information, in the footer. When processing is rule-based, an invoice number can be found when it matches a certain string, and a due date can be identified through the anchor “due on,” for instance.
If these kinds of rules for vendors, invoice numbers, etc. change, they must be re-configured in the software. When AI is involved, however, this manual step can be skipped. AI proceeds according to the basic principle we’ve explained, learning from patterns found in data. The more data you provide — in this case through the large volume of invoices — the more information the system ingests. As more information is processed, invoices that are new to the system are extracted more quickly and accurately based on recognized patterns. Imagine what a trained invoice-processing system might look like. It would probably have trained functionality for document separation, document capture, supplier recognition and the extraction of individual field contents. Then, if a system is AI-enabled, these functions can come together to automatically send a debtor a payment reminder based on the patterns recognized; or the system might let a user know that a certain part of the invoice was flagged for a probable recognition error.
From Field Identification to Document Separation
Via its AI functionality, the invoice processing software identifies information from recurring documents for automated invoice capture by drawing on the templates it created autonomously. In an iterative process, it learns from changes made when agents perform validation, continuously adapting the template. If, for example, a vendor changes its invoice layout, the software will recognize the changes and automatically adjust the template. If the finance department needs more fields to process a document, the employee who is handling the task can label the fields for the specifics required. The software will then recognize the new field and add it to the template.
Invoices are received by a company through different channels: by e-mail, by way of a portal, or through the traditional paper mail route. Documents might consist of one page or of hundreds. Everything that arrives in paper form must first be digitized. There are two approaches to separating documents. Both take into account the great variance in length, i.e., in the number of pages involved. With the first, each document is uniquely identified with a barcode sticker before scanning. The barcode indicates which page is the first page of an invoice, which enables the capture software to separate the documents correctly.
In the second approach, documents are separated manually once they have been digitized. Here too, AI can assist by independently identifying differences between new documents based on divergent layouts, page numbers, and data fields. When automated validation has been completed, manual corrections can still be made. The software solution in turn learns from these changes, draws on the training effects for future recognition, and derives new rules from them.
Critical Issues
Over the past decade, AI has developed in leaps and bounds. A broad range of practical applications has been developed, and its potential is far from being exhausted. Cheap computing capacity and a sufficiently large number of digital datasets fuel the use of machine learning and deep learning. The software industry is constantly evolving its products and, through the integration of AI, enabling them to intelligently and autonomously meet challenges in today’s business world.
A major focus is to relieve employees so that they can concentrate better on their core tasks and processes. Despite all the enthusiasm about what continues to be significant potential of AI in practice, however, the subject warrants critical examination as well. There are certainly ethical concerns, and rightly so. What would happen, for example, if machines were given the power to decide on issues of privacy or human self-determination? In the present day and age, it is critical to refrain from expecting that an artificially created intelligence might have the capacity to take on true responsibility.
We have not yet created a strong AI that is able to act flexibly, on its own initiative, with comprehensive thought processes. All technologies that are deployed at the moment only work with weak AI, where processes clearly define subtasks according to syntactic patterns and offer speed and precision as advantages over human performance. We can summarize by saying that an AI application can only do what it was designed to do. It is a tool and cannot think for you. For this reason, there are a few things to keep in mind when using an AI application:
- Legal and ethical aspects: Who is responsible if the AI-enabled software makes an incorrect decision (for example, in the event of accidents that occur in conjunction with autonomous driving)?
- Risk of bias: AI learns from the data it receives but does not question it. If a data set exhibits a bias, the algorithm will learn this bias. If the data set is not evaluated and adjusted for the bias, it can lead to discrimination in cases as we have seen in the use of AI in recruiting processes.
- Explainability and trust: AI derives decision-making criteria from the data set by itself. This is what makes AI applications something of a black box: It is not apparent to the user why a decision was made this way or that, which can be an issue of trust. One solution to this could be to have the application only make suggestions and to leave the actual decision to a human being. These types of decisions, sometimes also referred to as “algorithm-based decisions,” do not encroach on the self-determination of humans. In contrast, “algorithm-generated decisions” are created by algorithms and tend to transfer more autonomy to the system.
- Complexity vs. simplicity: Because it derives its own decision-making criteria, artificial intelligence can be used in complex contexts, i.e., when decisions cannot be made on the basis of simple if-then rules. Conversely, however, a fixed set of rules can be a much more efficient solution for simple questions. Adequate consideration should be given to these different types of contexts when applying software with AI.
AI Does Not Suit Every Business Process
Artificial intelligence is not an all-purpose tool for automating business processes; some areas of application are well-suited, but some simply are not. If AI is a hammer, it doesn’t mean that every business process is necessarily a nail.
Invoice processing is no exception: some scenarios profit from the use of AI technology, while some are less suited. Consider the step for determining the document type: The decision as to whether the invoice is FI or MM can be broken down to the single yes/no question, “Is there a purchase-order number on the invoice?” This simple task is perfectly suited for a fixed set of rules, and using AI at this point would be overkill.
Another example is agent determination: In companies with structures that have grown over many years with minimal documentation of processes and responsibilities, storing the data that specifies which employee is responsible for which documents and work steps, under which conditions, is labor-intensive, to say the least. This is where artificial intelligence can save time and effort. An alternative would be to store the decision-making logic manually, especially in the case of agent determination, which involves legal aspects with regard to invoice release. Agent determination can be clearly defined and derived through rulesets stored in the system. AI cannot always provide 100% security in this area. It is therefore important to weigh whether full automation can really be achieved with AI algorithms.
When it comes to document extraction, the content of an incoming invoice is largely prescribed by legal regulations. However, the layout is not. Even if the differences are subtle, they remain differences nonetheless, and these differences result in seemingly infinite variations on how a bill might ultimately appear. Imagine manually training a system with documents based on hundreds or thousands of suppliers, labeling the documents to indicate where different pieces of information are located. The limits of manual differentiation are obvious. It is clearly a time-consuming activity which could profit immensely from AI support.
And now let’s talk about account assignment for invoices: Specifying all the rules in advance is arduous if not impossible. Even if you manage to specify all existing scenarios, you won’t cover the ones that will materialize in the future. This is where a system-generated list of suggestions can come in handy. Such a list might be AI-based or may simply show the history of recent account assignments, both of which are helpful. If AI is used, you need to know what information is needed to find the correct account assignment. For example, recurring items such as G/L accounts or cost centers are better suited for automation than more one-time or temporary items such as SD orders or projects.
The Bottom Line: How to Maximize Benefit with AI
In the classic business process of invoice processing, artificial intelligence has enormous potential to ramp up efficiency — if it is used in the right places. When automating processes through AI, one must always consider what information is necessary, and where the information comes from. In many cases, the historical data available in the ERP system needs to be supplemented with additional information that may or may not be available in a machine-readable format. If you are able to work within the constraints of AI-enabled software, you’ll be rewarded with an incredibly useful tool for simplifying and automating operations and processes. AI can take your business processes to the next level.
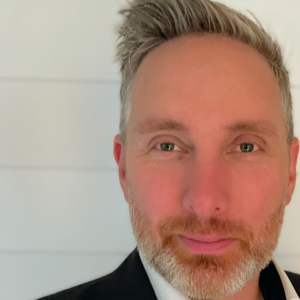
The post Artificial Intelligence in AP Automation – A Look at What Really Works, and What Doesn’t appeared first on Datanami.
0 Commentaires