Most of us want first-class treatment when we deal with a company, whether it’s on the Web, over the phone, or in person. We want them to know who we are and what we’ve done, and anticipate what we will need next. In the past, delivering this level of service was prohibitively expensive, simply because it required a ton of manpower. But in the age of big data, the clues to hyper-personalization are everywhere–if you know where to look.
The key to enabling hyper-personalization is to know as much about your customers as possible. That means collecting lots of data about your customers and their preferences. However, that poses a problem to most companies, says Paul Reiner, the vice president of digital transformation and innovation at Sutherland Global, a San Francisco-based provider of AI-based brand experience and call center services.
“The biggest limit right now is the company’s ability to collect data. That’s the biggest challenge,” Reiner tells Datanami. “Most companies do not have a very good, tight system for collecting omni-channel data and integrating it. They generally are okay with collecting it from any one channel. But figuring out how to integrate that together, how to handle the dedupes and create one form of identify for every consumer–that gets challenging and hard.”
The death of third-party cookies has hurt companies’ ability to collect data about their clients and prospects, but the good ones are finding ways to work around it, Reiner says. In some cases, they’re starting with data gathered from customers that gave their consent, and then using those to build “lookalike” customers that can be applied to other customers with similar profile and preferences.
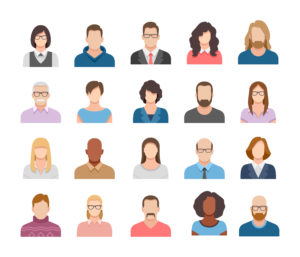
The lack of customer-level data means most companies are stuck using a handful of personas (kuroksta/Shutterstock)
However, that is clearly not hyper-personalization. The reality is, most companies are still in the segmentation phase where they use a handful of personas to represent their entire customer base. According to Reiner, many of the large companies that Sutherland works with have anywhere from 100 to 1,000 segmentations. “It’s a small segmentation,” he says. “Over time, you can get closer to one to one.”
The closer a company get to that one-to-one goal–which is true hyper-personalization–the better the results will be. Whether it’s offering a customer a discount on a concert they are likely interested in, recommending a hotel room that the client may find enticing, or satisfying a cellular phone issue that could result in customer churn, the potential for data-driven personalization to delight a customer or solve a problem cannot be ignored.
“Personalization is kind of a buzzword,” Reiner admits. “When you think about it, everybody is doing personalization to some level. Whether it’s calling you by name, realizing that you’re calling about a specific matter, realizing that you called before with the same phone number. There are some basic things. But then you get to much more sophisticated personalization, and that’s where we’re really trying to go with a lot of our clients.”
Knowing where to look for actionable data is the key to hyper-personalization, and Sutherland certainly knows where to look. Since spinning itself off from Xerox 36 years ago, the company has become one of the largest operators of call centers in the world. Many of Fortune 1000 companies outsource their call center operations to Sutherland. Across this communication channel and others, Sutherland has access to six million customer interactions per day, which it uses to drive hyper-personalization for its clients.
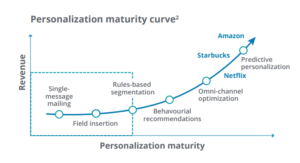
Where are you on the personalization maturity curve? (Image courtesy Deloitte report “Hyper-personalizing the customer experience using data, analytics, and AI”)
In the old days, customer service representatives (CSRs) might have been encouraged to take notes of their conversations with customers. Beyond addressing the matter at hand–such as dealing with a billing issue for an Internet service provider or signing up for a new account with a credit card company–the CSR might have learned additional information about their customers during their conversation, such as their hobbies and interests, or something about their friends and families.
If the CSRs were diligent notetakers, this additional information might have found its way into the customer relationship management (CRM) system in a useful manner, where it could be used to build a profile of the customer for future uses. But this approach rarely worked, says Doug Gilbert, Sutherland’s CIO and CDO.
“If I have humans do it, they’re just writing ridiculously vague notes that are not usable,” Gilbert says. “Ninety-seven percent of the data [shared by the customer during the telephone conversation] is thrown away. It’s ignored.”
Instead of letting all that potentially useful information fall by the wayside, Sutherland devised an automated method to capture it and turn it into something useful. It starts with recording every conversation between a customer and a CSR, and storing the conversation into 15-second “chunks.” The sound files are then turned into digital forms using a speech-to-text engine, and then natural language processing (NLP) techniques are used to extract meaningful information from that text.
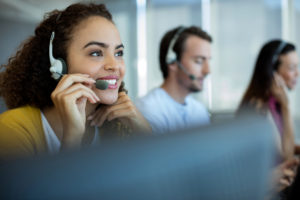
Transcripts of customer service calls is a rich source of data for hyper-personalization (wavebreakmedia/Shutterstock)
Named entity extraction (NER) and topic modeling are some of the techniques that it performs on conversational data sitting in its data lake. NER allows Sutherland to identify and associate customers with other people, products, places, dates, times, and other entities. The company also runs sentiment analysis models on the data, enabling it to automatically determine how the customers are feeling.
The information hidden in these conversations plays a big role in enabling hyper-personalization for Sutherland’s clients. In addition to enabling its clients to refine and improve the chatbots that they’re increasingly using to automate customer interactions, the insights derived from the conversational data can also be used to deliver real-time recommendations to human operators, Gilbert says.
“All this relevant information is typically communicated, just never captured,” Gilbert says. “We’re looking at every conversation. We’re analyzing 100%, not just 3%. Then, even from those conversations, we’re extracting 97 times more information than a human could.”
The company uses a variety of technologies to accomplish this, both on prem and in the cloud. It uses a popular open source Python library called spaCy for NER. It also works with Google Cloud, both as a customer for Contact Center AI and as a partner in developing its new natural language understanding (NLU) technology.
“We are one of the five co-developers of Google’s next gen NLP engine,” Gilbert says. “That thing is an evolutionary jump over what exists in the public today, which is a real-time NLP engine. This is NLU engine which brings complex understanding at the same time.”
All this AI powering hyper-personalization requires a sizable investment in network capacity and hardware. Sutherland operates in 27 data centers around the world, many of them co-located with hyperscalers, as it continually processes the conversations that its 50,000 CSRs have with clients, looking for actionable data.
“I move more data per day than Twitter moves in a year. It’s part of the nature of the beast,” Gilbert says. “We use a lot of TPUs back at Google. We have massive Nvidia GPU farms. We’re continually adding more. Right now, we’re backlogged about 1.5 million chunks, so we’re looking to continue to scale.”
With nearly 7 PB of data in its data lake, Sutherland is no stranger to big data. With access to some of the most sophisticated NLP and NLU technology on the planet–not to mention the fact that billions of conversations flow through its call centers every year–the company is on the cutting edge of turning those customer conversations into actionable data that gets its customers closer to that hyper-personalization dream.
Related Items:
Hyper-Personalization and AI Critical for Growth in Financial Services, Study Says
Leveraging AI to Deliver a Personalized Experience in the New Normal
Journey Analytics: A Killer App for Big Data?
The post In Search of Hyper-Personalized Customer Experiences appeared first on Datanami.
0 Commentaires