Just as computer processing power increase over time, we’ve come to expect that technology will successfully automate more complicated tasks every year. This is true in some digital domains, but this linear form of thinking could get you into trouble in the field of data science and machine learning, particularly when trying to replace full-blown data scientists with so-called “citizen” data scientists.
Data science is a complex field that requires practitioners to be proficient in multiple domains. While definitions abound, the prototypical data scientist is usually said to possess skills in three areas: computer science, mathematics/statistics, and domain knowledge. You have undoubtedly seen the Venn diagram that shows the overlap of these three domains, with the rare data scientist sitting right in the middle. Folks with this combination of skill are hard to find, which is why the average salary range for a data scientist nationally is $123,000 to $152,000, according to Salary.com.
There are variations on this definition–check out Turing Award winner Jeffrey Ullman’s thoughtful take on what is a data scientist here–with some tweaks and additions to the specific skills that a data scientist is said to require. Different industries use data science differently, which impacts the specific skills that are needed. There was a push several years ago to dilute what the title mean, with SQL-loving data analysts trying to usurp the title to qualify for more jobs and move up the salary range. But thankfully, that analyst broadside appears to have fallen by the wayside, and all in all, there appears to be somewhat broad agreement today by both employers and employees on what constitutes a data scientist.
That brings us to the topic of citizen data scientists, and whether they can actually replace their non-citizen brethren, or whether it’s just another case of trying to change the definitions of words in an attempt to thwart hostile reality.
One argument says that the growing sophistication of data science and machine learning platforms is decreasing the need for full-blown data scientists. As AutoML and other tools automate some of the tasks that used to fall to the data scientist–such as data preparation, feature and model selection, and hyperparameter tuning–some make the case that folks with a lower degree of skills and experience, i.e., the citizen data scientist, can drive the data science project successfully from start to finish.
That idea is getting significant pushback from experts in the field. One of those is Victor Thu, the president of Datatron, an MLOps tool vendor. While the citizen data scientist may find success using low-code AutoML tools to drive data science projects in some fields, Thu says, their lack of statistical mastery tends to lead them straight into a brick wall when it comes to one critical aspect of data science: the data.
“It is a bunch of marketing,” Thu says of the idea that AutoML tools can eliminate the need for a full-blown data scientist. “If you’re trying to design an AI or ML project or solution that works for the company, it’s not as simple as just throwing your data to an automated tool to come up with some results. It doesn’t work that way. You really need someone who has the appropriate training looking at data and understanding what the data is telling you, so that you can build a solution that is appropriate.”
One big hurdle that organizations run into time and time again is the state of the data. In short, the data often is a total mess, which is why data scientists spend so much time cleaning historical data before analyzing it for trends and, ultimately, training machine learning models to make predictions on new inputs. While data cleaning tools that can accelerate this task with automation have made big strides in recent years, there are still significant data quality gaps that will quickly trip up a data science project.
“A lot of the problems today [comes from the fact that] a lot of the data we have is not well curated,” Thu tells Datanami. “If the people who are giving it the data know what the data is about, chances are the AutoML will provide good enough results. But if it is a person who is not a trained data scientist, who basically just gathers a bunch of data maybe from their existing database,” then chances are the results will not be good.
Another perspective on the topic comes from Kjell Carllson, who is head of data science strategy and evangelism at Domino Data Lab.
“The ‘citizen data scientist’ (CDS) concept was invented with good intentions–to promote data, machine learning and AI literacy–but it has done more harm than good,” the former Forrester analyst writes in a recent blog piece on the Domino Data Lab website. “The pipe dream of an enterprise consisting of CDSes inevitably leads to expensive initiatives that, at best, lead to one-off insights that in most cases, have nothing to do with data science. Many companies that take this approach end up with nothing to show for it.”
Instead of trying to replace real data scientists with citizen data scientists, Carlsson wants “honorary” data scientist to help with some related data science tasks, such as data prep or data analysis. But when it comes to building the machine learning models, don’t try to replace a real data scientist with a citizen one–unless you enjoy getting calls from regulatory agencies.
“These essential, mission-critical–often regulated–models cannot, and should not, be created by anyone other than professional data scientists for the same reason that a hospital should not be staffed with ‘citizen surgeons, airlines should not rely on ‘citizen pilots, towers should not be built by ‘citizen architects,’ and your C-suite should not consist of ‘citizen managers,’” he writes.
Maybe we just need to rethink what a citizen data scientist is. Instead of functioning, in effect, as a junior data scientist, with all the responsibilities that come with being a full data scientist, perhaps citizen data scientists have a completely different set of responsibilities. That’s in effect the approach promoted by Gartner, which defines a citizen data scientist as “a person who creates or generates models that leverage predictive or prescriptive analytics, but whose primary job function is outside of the field of statistics and analytics.”
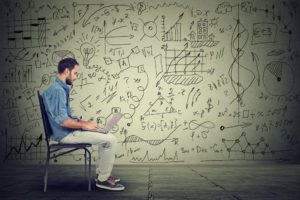
Data scientists should have at least a Master’s degree in a related field, Datatron’s Thu says (pathdoc/Shutterstock)
“The biggest struggle organizations face is the lack of clarity of responsibilities of a citizen data scientist,” Anirudh Ganeshan, an associate principal analyst at Gartner, says in a June 2021 blog piece on the Gartner website. “This vagueness creates hostilities among expert and citizen roles and impedes healthy collaboration and communication.”
While citizen data scientists may be able to help with some data prep and data analysis tasks, it should be done under the watchful eye of a real data scientist, who have the required mathematics and statistics training to avoid bad outcomes, Ganeshan says.
“Citizen data scientists must not work in isolation,” he says. “Citizen data scientists should not leverage self-service data science platforms in a siloed manner. Instead, they should participate in the development process with the expert data scientist who will eventually be responsible for validating those models before moving them into production.”
While schools have ramped up their data science education and training, there is still a substantial gap between supply and demand for data scientists. It’s tempting to try to replace expensive and hard-to-find data science unicorns with folks with the “citizen” title. Thu has a word of advice on that: don’t.
“If you want to build a good model, the last place you want to cut cost is on the data science side,” he says. “Because if you don’t prepare your data, prep your data well enough from the initial level and you just have a citizen data scientist do it, you’re actually going to incur a lot more costs down the pipeline because of potentially regulatory compliance issues that you may suffer downstream.”
Related Items:
What Is Data Science? A Turing Award Winner Shares His View
‘Data Scientist’ Title Evolving Into New Thing
Are We Asking Too Much from Citizen Data Scientists?
The post The Limits of Citizen Data Scientists appeared first on Datanami.
0 Commentaires