Foundation models like GPT-3, BLOOM, and BERT have garnered much attention as of late, for good reason. These versatile models, often trained with a vast amount of unstructured data, have immense capabilities that can be adapted to multiple applications, but their homogenous nature can sometimes allow defects to be passed from one to the next.
To shed light on these less understood models, The Center for Research on Foundation Models (CRFM) at Stanford University has developed a new benchmarking approach for large language models called Holistic Evaluation of Language Models (HELM). CRFM scholars have benchmarked 30 language models across a core set of scenarios and metrics under standardized conditions in order to highlight their capabilities and risks.
Intended to serve as a map for the world of language models, HELM will be continually updated over time with new scenarios, metrics, and models through collaboration with the broader AI community, according to CRFM researchers.
The team has highlighted its holistic approach, emphasizing how assessing language models in their totality is necessary for building transparency and achieving the more comprehensive understanding needed to improve and mitigate the societal impact of this technology. The team lists the following three elements of this approach:
- Broad coverage and recognition of incompleteness. Given language models’ vast surface of capabilities and risks, we need to evaluate language models over a broad range of scenarios. However, it is not possible to consider all the scenarios, so holistic evaluation should make explicit all the major scenarios and metrics that are missing.
- Multi-metric measurement. Societally beneficial systems are characterized by many desiderata, but benchmarking in AI often centers on one (usually accuracy). Holistic evaluation should represent these plural desiderata.
- Standardization. Our object of evaluation is the language model, not a scenario-specific system. Therefore, in order to meaningfully compare different LMs, the strategy for adapting an LM to a scenario should be controlled for. Furthermore, we should evaluate all the major LMs on the same scenarios to the extent possible.
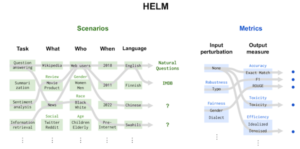
Under the HELM benchmark, models are evaluated across a core set of scenarios and metrics under standardized conditions. Source: Stanford University
The researchers ran over 4900 evaluations of different models on different scenarios, amounting to over 12 billion tokens of model inputs and outputs that spans 17 million model calls. Notable findings include how there are consistent performance disparities present in all models, including racialized dialect disparities: “OPT (175B) is the most accurate model on TwitterAAE, but its accuracy degrades from 1.506 bits per byte for White English to 2.114 bits per byte for African American English (lower is better).” The team also found that biases and toxicity in model generations are largely constant across models and are low overall for core scenarios. Additionally, accuracy consistently improves as models become larger, but it comes with higher training and inference costs. The researchers detailed their findings in a scholarly paper available here.
The researchers say that for full transparency, all raw model prompts and completions are released publicly for further analysis. A general modular toolkit is also available for adding new scenarios, models, metrics, and prompting strategies.
To read a blog post with more technical details of the HELM benchmark, visit this link.
Related Items:
Experts Disagree on the Utility of Large Language Models
Stanford Researchers Detail New Method for Error Detection in Perception Data
BLOOM Large Language Model Breaks Down the Walled Garden
The post Stanford Researchers Develop HELM Benchmark for Language Models appeared first on Datanami.
0 Commentaires