Despite the availability of better tools and technology, the quality of data is getting worse, according to a new report issued by data availability provider Monte Carlo. The instances of bad data taking an application or a company offline, or data downtime, is also trending in the wrong direction, Monte Carlo says.
As the fuel for digital commerce, data is a precious commodity that businesses cannot do without. Data engineers build pipelines to pump data from its source to its destination–such as a cloud data warehouse or a data lake–where it’s used to power analytic dashboards, for AI-powered automation, machine learning training, or to drive data products.
However, keeping those data pipelines running smoothly can be difficult. Problems in the data can arise through multiple means, including changes in data schema, data drift, data freshness issues, sensor malfunction, and manual data entry errors.
Monte Carlo, which develops observability tools to help detect data quality problems, commissioned Wakefield Research to look into how companies are coping with data quality. The survey of 200 data decision-makers (non Monte Carlo customers) was conducted in March of this year, and the results were released today with the second annual State of Data Quality.
At a gross level, the number of data incidents is going up. According to the 2023 edition of the data quality report, the average number of monthly data incidents went from 59 per organization in 2022 to 67 in 2023.
The percent of respondents spending four or more hours resolving data issues when they’re detected also increased from about 45% in 2022 to around 60% in 2023, with the average increase per organization increasing from nine hours per year to 15 hours per year, the report showed.
The report also shows that data problems are being caught more rarely by members of the IT team. The percentage of survey respondents saying data problems are found by business decisionmakers or stakeholders all of the time or most of the time increased from 47% last year to 74% this year.
When you add it all up, it shows that things are not getting better, says Lior Gavish, Monte Carlo’s co-founder and its CTO.
“Basically people are having more issues, spending more time on them, and generally getting into situations where their stakeholders and business are impacted before they can actually respond and fix things,” he says. “That’s probably not where we want to be as an industry.”
Having a zero-error environment isn’t feasible, Gavish says. In every human endeavor, there will be flaws. However, data errors have a very real impact on business. The key, then, is to devise a way to detect these data problems as soon as possible and to minimize their impact on customers.
Data Downtime
Monte Carlo also spent some time and space in the report exploring a relatively new idea, data downtime. The measurement of application and server downtime is well-trod country in the IT business. The idea of data downtime is similar, with some special caveats.
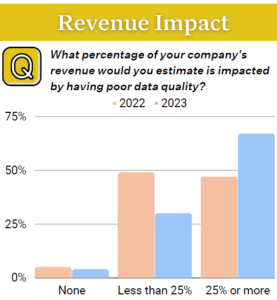
Data quality issues are having a bigger impact on organizations (Graphic source: Monte Carlo’s “State of Data Quality 2023”)
The company uses three metrics to measure data downtime, including time-to-detection, time-to-resolution, and the number of issues over time, according to Gavish.
Time-to-detection is a critical measure of awareness. “The longer an issue lingers in the system, the more problems it creates,” the CTO says. “Ideally, the data team wants to be able to find out about it on its own rather than getting stakeholders sending angry emails or Slack messages or calls or whatever it is.”
Time-to-resolution is important for measuring responsiveness to problems. “When something happens, there’s a big difference between you being able to fix it within minutes versus you chasing it down for week, both in terms of how long the problem persists and the impact on the business, and also in terms of how much engineering resources you’re throwing into solving this problem,” Gavish says.
Tracking the raw number of issues is also important, with lower obviously being better. “You can’t get down to zero,” Gavish says. “There will always be issues. But there are certain things you can do in terms of adding visibility and improving communications within teams and providing analytics that can help actually reduce the number of times something happens.”
Taking Responsibility
Data errors increase as a natural byproduct of the growth in data and data products, according to Gavish. As companies use more database tables and dbt models, the complexity increases exponentially because of the need to correlate each table or model with all the others.
Data testing using a tool like dbt or Great Expectations can help detect some of these errors. The Monte Carlo survey found that companies had an average of 290 tests. However, there are limits to what testing can do, since humans can’t anticipate every way that errors will arise.
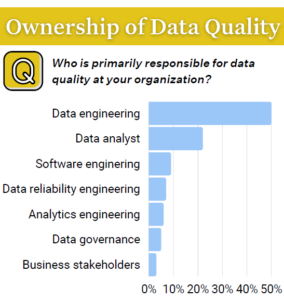
Data engineers are often in charge of data quality (Graphic source: Monte Carlo’s “State of Data Quality 2023”)
When data problems do rear their little heads, finger-pointing is a natural response. Because human error is often the culprit of data errors, somebody usually is to blame. But instead of casting stones, a better approach is to have clearly delineated roles and a good path of communication to resolve problems quickly.
The Monte Carlo study shows that data engineers are, far and away, the primary owners of data quality at organizations, with a 50% share among survey respondents. That’s followed by data analysts at just over 20%, with the remainder of the responsibility being spread out among software engineering, data reliability engineering, analytics engineering, data governance, and business stakeholders.
Having data engineers in charge of data problems has its strengths and weaknesses, according to Monte Carlo. On the plus side, they’re well-equipped to handle system-wide problems, the company says. However, they tend to have limited domain knowledge. “The reverse tends to be the case with analysts,” it says.
Unfortunately, the data quality trend is not pointing in a good direction at the moment. Will it get any better? Gavish seems to have his doubts.
“We kind of hope to see things gettering better, and they’re not, and the reasons are, as far as I can tell, we’re building larger data teams, we’re building more data products in general, and…the level of complexity and the number of things that can go wrong actually increases. So we’re just seeing more issues happening.”
Related Items:
We’re Still in the ‘Wild West’ When it Comes to Data Governance, StreamSets Says
What We Can Learn From Famous Data Quality Disasters in Pop Culture
The post Data Quality Is Getting Worse, Monte Carlo Says appeared first on Datanami.
0 Commentaires