What’s the next generation of enterprise AI going to look like? If you ask DataRobot CEO Debanjan Saha, enterprises will see the most business benefits by combining new generative AI tools and techniques with the classical AI and machine learning approaches that customers have honed over the past decade.
Saha, who joined DataRobot as president and COO about 18 months ago, brings a long track record of building enterprise data products for some of the biggest companies on the planet, including Google Cloud, where he was VP and GM of the data analytics group, and Amazon Web Services, where he oversaw Aurora and RDS.
Saha brings a no-nonsense engineer’s perspective to the chief executive’s office, which he has occupied since the beginning of July 2022. During the past six months, he’s embarked upon a whirlwind tour that had him visit 100 customers in 20 cities around the world. That tour has been quite informative, especially when it comes to generative AI and large language model (LLM) technologies such as ChatGPT.
“They’re all excited about it,” Saha told Datanami in a recent interview. “They’re anxious about it because they know that their board is asking about it. Their CEO is asking about it. I’m talking to the board members and the CEOs and they’re trying to figure out ‘Okay, this is great, but I mean, how many chatbots are we going to make?’”
There’s no denying the impact that ChatGPT has had on the field of AI. After all, we’re living through AI’s iPhone moment, Saha said. After years of struggling to find a way to successful work machine learning and other forms of AI into the enterprise, ChatGPT has put AI on people’s map in a big way.
“A lot of people thought of AI as one of these novel, esoteric technologies. They didn’t quite understand what AI can do,” he said. “Now everybody does, right? And that has kind of changed the momentum.”
Unfortunately, when it comes to actually delivering business value, there’s no real “there” there with the latest round of Gen AI and LLM technology, at least not yet. “I think we’re a little ahead of our skis,” Saha said.
While Saha is grateful that advances in AI are finally getting the wider recognition they deserve, there’s still quite a bit of work to do to fully integrate it into the enterprise.
“In my view, I think the proof is going to be in the pudding,” he said. “All the euphoria is going to last for so long. Ultimately, the business needs to show value from AI, and generative AI is no exception. Otherwise, we are going to be the same situation we have been with AI right now.”
The problem is that the track record for traditional machine learning and what he termed “classical AI” is not great. There are numerous studies showing that only a small number of enterprises (usually the larger ones) have been able to reap the rewards from AI and ML. Most have been stuck in mud, with questionable data and haphazard processes around the ML and AI workflows.
“AI has been around for a very long time and people have been using AI in various different ways and various different places for a long time,” Saha said. “To tell you the truth, in my view, it hasn’t really lived up to the expectation with respect to creating business impact that people thought AI can create.”
While Gen AI and LLMs have basically broken the hype meters over the past eight months, they won’t solve the AI struggles enterprises have gone through over the past 10 years. That doesn’t mean they don’t have value. But according to Saha, generative AI apps built on LLMs will comprise perhaps 10 to 20% of the overall AI solution.
“What I’ve seen is people taking generic LLMs and making them more subject matter experts in specific areas,” he said. “Everybody will have Langchain and they’ll figure out how to use that data to either fine tune the model or in many cases use a nice prompting strategy to make them more knowledgeable about a specific area.”
But that’s not where the real action is going to be, he said. “That’s a component. [But] I think ultimately it’s going to be combination of generative AI and predictive AI and finding the right use case, doing the right problem framing, and then figuring out where the ROI is going to be from this,” he said.
The bulk of the action in successful enterprise AI strategies, Saha said, will involve a lot of hard work. It will involve mapping AI tech to the specific business problem that the enterprise faces. It will require building a robust data pipeline to feed models. And it will require creating resilient workflows to handle the training, deployment, and monitoring of the AI models. And lastly it will require integration with the rest of the business processes and applications. In short, all the same stuff that has tripped up classical AI adopters for the past decade.
While the AI tech has advanced, there won’t be any shortcuts to doing the work of i ntegrating it into the enterprise, Saha said. The hyperscalers will provide some solutions, but they’ll lock you into their cloud and they’ll also require technical skills to integrate the pre-built components into your specific environment.
Enterprises will be able to buy off-the-shelf AI apps from vendors, but they will be of limited value since they will only focus on a specific area. “It is covering only one use case, and if you want to cover everything that you do in the enterprise, [you’ll need] maybe couple of hundred of those in order to build the entire folio, which is not an easy thing to do either,” Saha said.
Naturally, Saha sees a large opportunity for DataRobot and other vendors in the AI space who can help enterprises connect the dots and build end-to-end AI solutions.
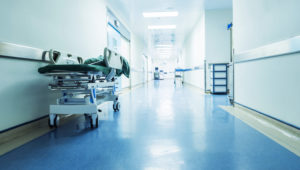
A hospital uses DataRobot’s generative AI capabilities in concert with predictive AI (hxdbzxy/Shutterstock)
“Our strategy has been–and this is what DataRobot has done successfully with classical AI–is, how do you make it easy for people to get value out of generative AI? And not just generative AI, but generative AI and predictive AI together?”
While the DataRobot platform was originally built for predictive AI, the company is actively morphing it to handle new generative AI use cases. It won’t require major tweaks, Saha said, because many of the AI processes that DataRobot has already automated for predictive AI—from data prep to model monitoring–can be used for generative AI workloads, too.
Many of the LLMs that enterprises want to use are open source and available from sources like Huggingface and GitHub, Saha said. And if a DataRobot customer wants to tap into GPT-4 or other LLMs from Google, they have the option of using APIs within the DataRobot platform, he said.
To help customers understand how the various LLMs are running on their data, DataRobot will deliver a leaderboard. That product is currently under development, and could be announced next month, Saha said.
Saha sees the combination of predictive AI and generative AI paying dividends for his customers. In many cases, generative AI functions as the “last mile,” connecting the customer with the insight generated from the predictive AI.
For example, one of DataRobot’s customers uses predictive AI model to determine whether a specific customer is likely to churn. When the model spots a customer that fits the profile, it triggers a generative AI workflow that sends a customized email to the customer or surfaces a script to an agent to address the concern.
Another DataRobot customer uses the two types of AI in a hospital setting. The predictive AI model does the hard work of combining various data points to determine the likelihood of a patient being readmitted. Then the generative AI model takes that output and generates an English language explanation of the readmission calculation, which is included with the patient discharge paperwork.
“Those are the kinds of things that could be really, really interesting,” Saha said. “There are tons and tons of use cases of that type.”
DataRobot has about 1,000 customers, and it will be working with them to implement generative AI into their workflows. Smaller firms like DataRobot have a big advantage over cloud giants like Google and AWS as far as actually working with customers on their particular problems, as opposed to selling them a set of do-it-yourself “Lego blocks,” Saha said.
But the shift from purely predictive AI to a combination of predictive and generative AI will also help DataRobot target new customers who want repeatable AI processes instead of ad hoc AI mayhem. It will also allow DataRobot to target a new class of users, Saha said.
“I do think that’s going to increase the aperture in terms of the business outcome,” he said. “Its not just people who deal with data and data science–it’s a much broader section of user base who now will be able to generative AI and AI in general.”
Related Items:
Data Management Implications for Generative AI
Large Language Models: Don’t Believe the Hype
DataRobot Introduces Expanded AI Cloud Capabilities and Tools
The post DataRobot CEO Sees Success at Junction of Gen AI and ‘Classical AI’ appeared first on Datanami.
0 Commentaires