So the decision has been made to upgrade your business using generative AI technology. Great! But now comes the hard part: Figuring out how to transform that aspiration into action, and it all starts with people.
The first move you’ll make in your GenAI journey is assembling the group of folks who will design, build, test, and deploy your GenAI applications. While these projects often involve automating work done by humans, GenAI development is very much a people-centric journey.
Technical roles are critical in GenAI application development. Depending on whether you’re building your own AI model (unlikely), fine-tuning an existing model (a little more likely), or adopting a pre-built AI model with prompt engineering and RAG (most likely), your GenAI team will demand a mix of data scientists, AI engineers, data engineers, and application developers.
But GenAI is not the exclusive domain of the techies. In fact, compared to classical machine learning projects, the role played by non-technical practitioners is even greater.
Non-Technical Roles
You won’t get far without the necessary technical expertise in GenAI. However, when using GenAI to modify core company functions, you’ll need the input from line-of-business experts, such as the head of customer support or the director of warehouse management, to ensure that your GenAI product is a good match to how they see customers and employees interacting with your brand.
If your GenAI targets any critical outward-facing functions, such as customer service, you’ll need input from high-level executives, if not the board of directors itself, to ensure that the company’s values are being upheld and you’re not doing anything to embarrass it. Similarly, if any security or ethical concerns arise in a GenAI project, that’s not something you can leave to the Web developer or data engineer; you’ll need the expertise of a security professional or a trained ethicist.
The good news is that you don’t have to hire all of these folks
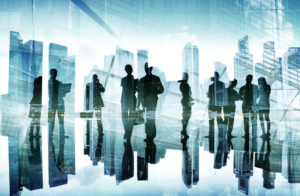
Non-technical roles are more important in GenAI than classical machine learning (Rawpixel.com/Shutterstock)
yourself. You can tap outside experts or tech consultants to help you build out your team. These consulting firms can provide much of the technical expertise that’s required, and depending on the size of the project, they may even be able to serve as the project manager to keep everything running smoothly.
One of the consulting firms that’s helping clients to build GenAI solutions is EPAM Solutions. The Newtown, Pennsylvania-based company employs more than 50,000 people around the world and is rapidly expanding its GenAI services team.
EPAM’s Rule of Three
EPAM uses a rule of three in constructing GenAI teams, says Pierre Samec, the company’s SVP of Enterprise AI Solutions. The teams are built out following a general rule:
“One is a product manager or business leader who decides what is the prioritization of the backlog,” he says. “One is a subject matter expert. And subject matter expertise is really fundamental in the GenAI space because if you don’t speak the language, it doesn’t work.
“The third role is what we call a GenAI builder, which is this person who can do prompt engineering but is also a full-stack engineer who can go fetch the data, fetch the APIs, fetch the services,” Semec says.
That triumvirate forms the core team of people, or the pod, that EPAM uses in GenAI client engagements. That doesn’t include outside expertise, such as security and ethics, which Semec views as horizontal structures within each organization.
The Two-Pizza Team
EPAM currently maintains 13 different pods that attack nine industry verticals (consumer processed goods, oil and gas, etc.) and four horizontal organizations (sales and marketing, warehouse management, etc.) he says. Each individual team usually contains between four and 10 team members, he says.
“It’s somewhere between a one- and a two-pizza team,” he says.
Small teams are often preferred when getting a project going, as they don’t require as much overhead and can be more agile. Online bookseller Amazon famously keeps its tech teams lean and mean by employing the “two-pizza” rule: If the team is so large that it requires more than two pizzas to feed them, then it’s too big.
Communication is just easier with smaller teams, said James Massa, JPMorgan Chase’s senior executive director of software engineering and architecture.
“You notice when there are a lot of people, communication gets [harder] and that’s why two guys in a garage always do better starting Apple than 100 people in a warehouse,” Massa said at the SolixEmpower conference in San Diego two weeks ago.
The Full-Stack CEO
GenAI tech is evolving at a furious clip, which is good news for your GenAI prospects. Tech giants like Google and Meta have gifted the world pre-trained large language models (LLMs)–you just need to tap into them and use them in a way that’s productive and profitable for your business, without sacrificing security and ethics along the way.
That availability of pretrained models lessens the need for you to hire a highly trained data scientist with the classical Venn diagram of abilities: One part mathematician and statistical wiz, one part computer science uber nerd, and one part business prodigy.
“Today AI is about creating behaviors of how you’re going to engage your customers, or how are we going to do something else, or how are we going to do this task,” EPAM’s Semec tells BigDATAwire. “So people who are good at ML … are okay. But you’ll see also see a new generation of younger full stack engineers who are very focused on behaviors that also are really promising in that space and broadening the spectrum of what can be done.”
The huge potential of GenAI also opens up the question of leadership. Traditional machine learning was more about optimizing some back-office function, but GenAI has the potential to radically transform your businesses relationship with its customers. Not every company will be able to withstand such massive upheaval.
“The question is what do you want to do?” Samec says. “Do you want to grow fast, damn the torpedoes, break [stuff], and see what happens? Or do you want to be mindful and proper, etc?” Samec says.
“I think leadership makes a huge difference,” says Samec, who oversaw the data transformation at Expedia in a prior role. “It’s actually not about that much about size [of the team] as much as the leadership level of the CEO to find differentiating factors in the areas of customer service, operations, go to market, and new product creation. It’s very early days on that side, but you can start seeing the first tremors of that happening now on the horizon.”
Related Items:
Tips on Building a Winning Data and AI Strategy from JPMC
GenAI Hype Bubble Refuses to Pop
What Is Data Science? A Turing Award Winner Shares His View
The post Building Your GenAI Dream Team appeared first on BigDATAwire.
0 Commentaires